Author: Dan James
Date: March, 2023
Text: PDF (https://doi.org/10.53975/1y7h-g9wd)
Abstract
There is an ever-increasing number of quantitative metrics, most of which are intended to act as proxies of quality for either authors or journals in current scholarly publishing. In contrast, this paper presents a more directly qualitative paper-level metric that adds a falsifiability dimension to the existing methods used to assess scholarly research. This new metric, the "F-index", is derived from a "Falsifiability Statement" (FS) (examples of both are applied self-referentially in Annex A). An FS is a discrete metalevel statement provided by the author(s) outlining how their research or assumptions can be foreseeably falsified, and the F-index is a numerical estimate of how clear and practical the steps are to falsify the research or stated assumptions as outlined in the FS. Though the F-index is particularly suited to hypothesis or theory-driven fields, it is also relevant to any empirical inquiry that relies on propositions or assumptions that can be potentially falsified. An F-index is qualitative in that a high F-index number provides a good indication of how novel or original a paper is. Four candidate mechanisms for obtaining an F-index from a Falsifiability Statement are evaluated: a peer reviewer assessed metric, an author or self-reporting metric, a propositional density metric, and an NLP derived metric. This evaluation concludes that a FS is currently a practical proposition, and that the derivation of a meaningful F-Index is an achievable goal.
“A theory with greater content is one that can be more severely tested”
– Karl Popper
1. Introduction
The naive falsificationist idea that a single incisive data point could falsify a whole research program has long been discredited; the actual practice of science doesn't follow simplistic rules or, arguably, any 'scientific method' (Feyerband, 1974). Research may be messy, motivations complex, and facts not so much uncovered as generated with multiple contributions (Latour and Woolgar, 1979). Methodological prescriptions may no longer constrain researchers for everyday work, but, as part of any critical review process an evaluative resource such as Popperian 'falsifiability' is still useful to qualitatively assess both research and researchers (Derksen, 2019). This paper explores the feasibility of a falsifiability tool built as an integral part of the scholarly publishing process -'putting Popper to work' to use Maarten Derksen's apt phrase (Derksen, 2019).
2. Scientometrics
Measuring and analysing scholarly output has become an intrinsic part of academic publishing with a recent proliferation of metrics–there are over 40 variants of the pre-eminent h-index alone (Rosy, 2020). Existing metrics attempt to provide quantitative measures interpretable as proxies for qualitative assessment, yet critics argue that an over-reliance on quantitative measures creates a system of perverse incentives and a publish-or-perish environment to the overall detriment of research (Thelwall and Kousha, 2021).
Instead of relying on metrics as proxies for quality, can a more in-depth metalevel discourse, based directly on qualitative rather than quantitative measures, improve research publication oversight? Current good practice guidelines already suggest including a 'limitations' commentary as part of a discussion section of research papers (PLoS, 2022), but could this be expanded to become a discrete (stand-alone) metalevel statement from which to derive a qualitative metric?
The authors of a paper know their data or research better than anyone, so it follows that they are best placed to provide a discrete statement regarding the falsifiable nature of the claims or propositions included in the paper - a 'falsifiability statement' (FS). From an analysis of a stand-alone FS, (as opposed to an analysis of the whole paper), a useful article-level metric–the F-index–can then be derived.
An FS is qualitative in the Popperian sense of evaluating propositions/theories against a stress test of falsifiability. Scholarly quality is a multidimensional concept resistant to description by any one metric (Moed, 2014), and a falsifiability tool would form part of an overall mix of metrics, altmetrics and peer review, yet bring specific advantages to the publishing process.
To derive an F-index metric from an FS, four candidate mechanisms are shown in the following conceptual flowchart (Table 1), and discussed in detail in later sections.
3. Metalevel Statement – the Falsifiability Statement (FS)
3.1 Precedents for an FS
Aside from the existing precedent of a recommended embedded limitations section for research papers (PLoS, 2022), there is also an example of a metalevel falsifiability statement in an area where the evidential process is similarly paramount – the criminal justice system. In the UK, the Criminal Procedure & Investigations Act 1996 explicitly requires police investigations to disclose, in the form of a discrete statement to the defence team, any evidence that would compromise or falsify the conclusions of their prosecution case. This formalises a falsifiability statement in a way that is easily transferable to existing processes of research publication.
A parallel to analysing an FS can be found in recent metaresearch which has used AI Natural Language Processing tools, (NLP), to examine and annotate a large corpus of peer reviews, establishing identifiable measures/qualitative dimensions and their possible correlation with journal impact factor metrics, (Severin, 2022). This work is similar in principle to analysing an FS, focussing an evaluation on a metalevel statement about a paper (in this case a corpus of peer reviews), rather than evaluating the paper itself. Both a peer review and a hypothesized FS fall into a broader category of metalevel statements, a category defined as a level of discourse about the object itself (paper).
3.2 Producing an FS
Each FS is individual to its referent paper, and how an FS is structured and argued is central in determining the quality of the FS and, by extension, the paper itself. Guidelines that suggest how to write an FS are similar to those recommended for writing an embedded limitations section. How the author’s attitude of critical reflection is expressed through the FS is what any subsequent analysis of the FS aims to capture or measure to derive the numerical estimate of an F-index. The following are some identifiable prerequisites of an FS:
Attitude:
Adopt a critical self-reflective attitude that concentrates on any weaknesses in the paper’s design, structure or data analysis and record this examination. Conduct and describe a rigorous scrutiny (of the research) based on identifying initial and concluding assumptions
Structure:
Enumerate the methodological limitations of the research and all falsifiable propositions
Enumerate all applicable data and cognitive biases
Commentary:
Explain in detail how each limitation/proposition could foreseeably be falsified
Explain in detail the steps taken to avoid data and cognitive bias and how these steps could be foreseeably falsified
Below is an illustration of how structure and clarity can be evidenced in the specific case of a confirmation bias workflow. In an exemplar FS a similar workflow for all other applicable cognitive biases would also be required (e.g., population bias, analytics bias, outlier bias).
4. Candidate mechanisms to produce the F-index
4.1 Reviewer-reporting metric – the F-index
An obvious way of deriving an F-index score is to allow reviewers to assign one after considering the FS (supplied by the author (s). This would put an evaluation of an FS on a par with an examination of pre-registered hypotheses, methods, data and findings that is an established part of the peer review process.
The difficulty with this approach is that heterogeneity amongst reviewers will almost certainly entail a further calculation of a mean numerical score, potentially raising a focus of dispute with the authors of a paper. In addition, an exclusively reviewer-derived F-index exposes the process to a risk of bias, for example, with the potential homophily amongst reviewers' interests that is claimed to introduce arbitrariness (Brezis and Birukou, 2020). To reduce the impact of bias, conceivably, a weighted average of reviewers' numerical estimates of an F-index together with an author estimate could be derived, with the weight attributed to the reviewers' aggregated scores or, in the alternative, the author(s). Which would be an optimal solution is an empirical question.
4.2 Author-reporting metric – the F-index
An FS is supplied, alongside an author-reported numerical estimate (for example, from 1-10), of the paper's falsifiability (as outlined in the FS) – an F-index. The two components taken together would mitigate against any misuse or misreporting in the following way:
Take the example of an author submitting a high claim for an F-index, given that a higher numerical estimate would indicate a high potential for falsifiability. So the author would be claiming that her research is highly falsifiable; however, this claim can be compared to her self-reported outline in the FS of how her research can be falsified or limited. If this outline is not a clear description of the steps required to falsify, or there is not an obvious way to falsify the research as claimed by the high F-index, this would be a mismatch, immediately calling into question the ethical basis of both claims and consequently the overall quality of the research.
The utility of providing a numerical estimate for the F-index is its ease of use when searching through (online) journals or repositories for just those papers that claim a high F-index, as these would be claiming the most falsifiable results. In addition, high F-index papers would be fertile ground for new ideas because a high F-index will be a signifier of highly falsifiable research, therefore an excellent topic to try to falsify or replicate and, if successfully falsified, potentially generate new hypotheses.
To take a further example: consider a paper that asserts the Earth is in fact a flat disc and not an oblate sphere, where the author is so sure of his claim that he has given it a high F-index to ensure maximum visibility in any online search of prized high F-index rated papers.
In such a paper there would be an expectation that the FS would have to outline with significant precision and clarity (because the F-index is high), the ways such a proposition could be falsified, in the absence of which it would be safe to assume the FS as a whole is misleading thereby invalidating the conclusion. (Of course, in this particular case, the author could indeed supply exact means to falsify his principal claim, and it would be trivially easy to use them to do precisely that). So the numerical F-index alone does not comment on the demarcation problem (of science from non-science), but, in conjunction with the FS, makes the demarcation clearer. High F-indexes would be sought after by authors and readers alike because they would signify a higher quality of propositional content. However, this higher content is proportional to a more severe author-devised test.
4.3 Propositional density metric
A proposition is an idea unit, a statement that expresses a claim and Idea Density or proposition density - P-density- is the number of expressed propositions divided by the number of words in a sample of text - a useful estimate of the complexity of embedded syntax. P-density refers to the amount of meaning conveyed in a text through the relationship between its various information elements (Brown, 2008), suggesting a well-constructed falsification statement (FS) is likely to contain a high P-density.
Whilst this might appear to be a straightforward method of deriving an F-index numerical value, research has shown that P-density alone cannot discriminate between different types of text (DeFrancesco and Perkins, 2012). It may be the case that a well-constructed FS has a high P-density in terms of the complexity of its syntax, but a simple P-density number fails to capture the semantic content of a text sample. Consequently, though a P-density analysis could be useful as an auxiliary tool in assessing the syntax complexity of an FS, it’s insufficient on its own to arrive at a meaningful F-index metric.
4.4 NLP derived metric
For text analysis, a P-density approach now distinctly underperforms compared to the present, rapidly evolving NLP and Large Language Model (LLM) ecosystem. Text analysis is a controversial topic in education with the emergence of increasingly sophisticated Automated Essay Scoring (AES) to the extent that it's now possible to foresee a dystopian circularity of AI-assisted essay writing graded by AES. Despite ethical concerns about implementation (Basbøll, 2022), AES research does provide valuable insights into a possible methodology for analysing an FS to derive a falsifiability metric.
Many AES systems attempt to score different dimensions of an essay's quality in the following way, (see Table 2, below).
Taken together, the dimensions shown in Table 2 are usually scored and presented as a holistic analysis of an essay, but feedback even on a particular dimension may not explain why the overall score is low, and so to address this concern, some researchers have developed language models that can explain why an essay receives a particular score along a given dimension based on a key overriding dimension of essay quality – argument persuasiveness (Ke, 2021).
Argument persuasiveness is a prime candidate for an analysis of an FS, but it is challenging to develop a language model for, requiring as it does a persuasiveness-annotated corpus to train a language model, a corpus as yet not available for any discrete FS. Nonetheless, it is instructive to see a possible scoring system (see Table 3, below), derived from the attributes the authors (Ke, 2018) have annotated in the corpora they have used and a summary of those attributes (see Table 4, overleaf).
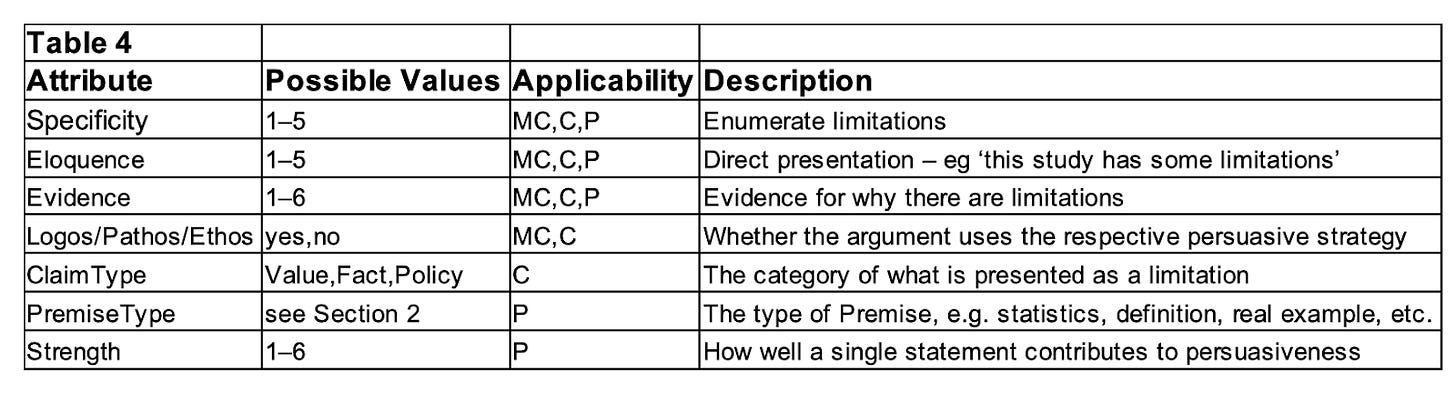
Based on Table 4 above, a proposed summary of attributes and possible scoring values can be constructed as the basis for a hypothetical FS metric – see Table 5 below.
Whilst the precise methods by which a language model can be trained/built based on the attributes shown in Tables 4 & 5, are beyond the scope of the present paper, the authors quoted (Ke, 2019), claim that they have indeed built such a model, though their goal is to make it more robust by continuing to improve attribute prediction.
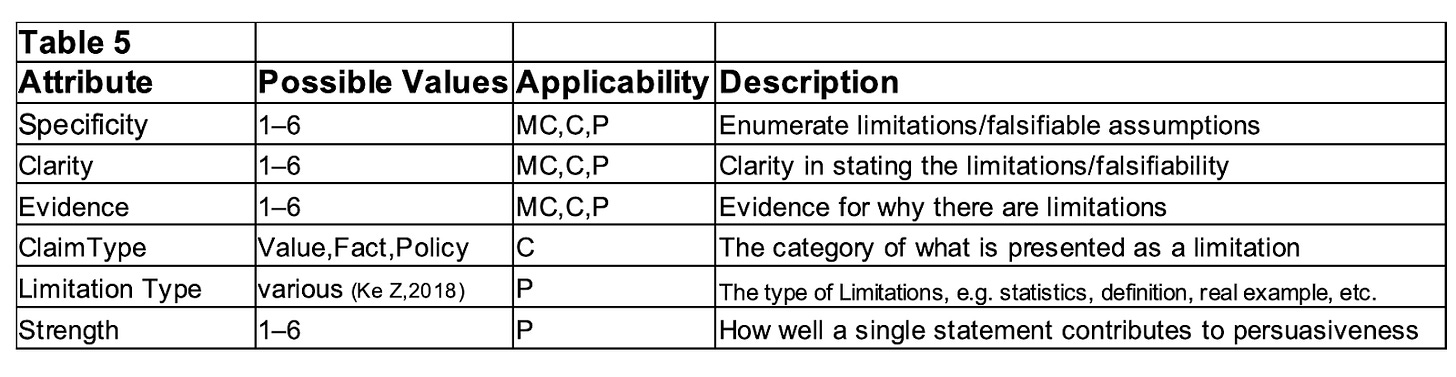
5. Metascience Discussion
There are two components to the present proposal: an FS, a discrete falsifiability statement, and an F-index, where the FS is conditional on a written paper. But an FS and an F-index do not necessarily both have to be implemented because a discrete FS is of utility, even without an F-index.
Would an FS and a subsequently derived F-index apply to all paper types? It is difficult to conceive of a publishable paper that contains no propositional claims, for in their absence, what would be the content or purpose? Even a paper with the barest minimum of propositions is a good candidate for an FS, as propositions are normally defined in science as statements reliant on reasonable assumptions and existing correlative evidence. Assumptions are a potent ground for introducing bias and preference, therefore questioning stated assumptions would be an important part of an FS.
Whilst it's tempting to think that an FS is most applicable in areas such as the 'hard' sciences where articles are most obviously hypothesis-driven or theory-driven (and therefore falsifiable), an FS is equally relevant to fields that deal with complex systems where empirical evidence is difficult to obtain, for example, sociology or economics, that also rely on propositions or assumptions that can be potentially falsified.
An FS is concerned solely with those propositions which it is feasible to falsify and the more practical it is to do so results in a higher F-index. So in an extreme situation, a paper could have a minimum number of propositions, yet dependent on their nature, still achieve a high F-index. Conversely, a paper with many propositions, few of which the authors can consider a means to falsify, would have a low F-index.
It's interesting to consider how papers at either end of an F-index scale would look. Would, for example, a highly innovative paper run the 'risk' of only achieving a mid-to-low F-index and consequently appear less visible in online searches for high F-index papers? Highly innovative papers by definition, contain propositions and claims that are novel or out of the ordinary, and a good heuristic is to require extraordinary claims to be matched by extraordinary evidence, (apologies to C. Sagan), which the authors can then outline possible means to falsify. The message here is that it is not falsifying per se that is the concern of an FS, but rather the clarity of reporting what reasonable steps it would take to falsify the results. All it takes is for these steps to be clearly explained to achieve a high F-index for an innovative paper.
6. Conclusion
This paper proposes an author-written falsifiability statement (FS), as the basis of an article-level falsifiability metric - the F-index. Good practice in writing research papers currently recommends an embedded limitations section. A formal requirement that a limitations statement is extended to form an FS, as a discrete part of a paper, is a practical possibility, supplying a focus for both authors and reviewers/readers on falsifiability as a functional epistemic and evaluative dimension, in addition to facilitating the creation of an F-index metric.
Four candidate mechanisms for analysing an FS to produce a metric were examined. First, propositional density measures alone cannot clearly distinguish between different texts and do not help in terms of a qualitative assessment. A peer reviewer-assigned metric or one derived in combination with an author self-reporting F-index, though initially appearing susceptible to misuse, bias and ‘gaming’, can potentially be helpful if always compared and contrasted against an FS. Finally, a language model trained on a dimension of argument persuasiveness and producing a measure of falsifiability is feasible. There are, therefore, good grounds to think that a meaningful F-index metric is an achievable goal.
Annex A
Note: To potentially obtain a high F-index the precise specifications and methods for qualitative attitude or empirical testing/research would need to be supplied.
F-index: 6.5
Falsifiability Statement
This paper has 6 identifiable limitations/ assumptions that could foreseeably be falsified.
1 - Value assumption: that an additional metric is required.
There are numerous metrics for both papers and journals so it is doubtful that another one would add anything, or be welcomed by any particular research community.
Falsified by: – qualitative attitude survey
2 - Definition assumption: falsifiability.
Falsifiability has a distinctly archaic ‘feel’ to it, the word ‘limitations’ is already used for essentially the same purpose. Alternatively use a ‘Bias’ statement, ‘Transparency’ statement or indeed as used in the paper itself -’Metalevel Statement’?
Comment: though admittedly slightly archaic sounding, falsifiability arguably better captures the nuances of the purpose it is used for in this paper, namely to outline means to disconfirm results.
Falsified by: – qualitative attitude survey
3 - Value assumption: Author-reporting metric.
A self-reporting metric even weighted against a peer reviewer estimation may be open to more misreporting and ‘gaming’ than envisaged here, making the resulting numerical estimate meaningless.
Falsified by: – empirical testing/research.
4 - Adoption assumption: F-index
An F-index to be workable would have to be adopted universally by all publishers/repositories with one standard way of deriving it. Given that there is no general agreement even on data standards and metadata standards at the moment, this seems unrealistic.
Comment: an FS is a practical possibility for a publisher to implement immediately and if it proved worthwhile would doubtless be taken up by others. The same reasoning essentially applies to an F-index. It’s true that if only one publisher instigates an F-index protocol it would not offer a realistic measure comparable to papers with no F-index. But this could change rapidly if an effective language model produces a reliable F-index. Conceivably different publishers would, to begin with, use their own language models to derive an F-index, with the most popular/effective one evolving.
Falsified by: empirical testing/research.
5 - Preferred attribute dimensions
Choice of argument persuasiveness as the key qualitative dimension for a proposed language model to establish an F-index is proposed by the paper but can we be certain that there are not other analysis dimensions equally valid?
Falsified by: empirical testing of proposed model.
6 - Statistical assumption:
A range of possible values for an F-index, even assuming that the values are realistic, would probably follow a normal distribution. So with most values being in the mid-range the distinctions between each value would be minimal and not useful to gauge the merits of any one paper.
Comment: It’s true that most mid-range values for an F-index would be hard to differentiate in terms of a proxy for innovation/novelty, but where an F-index is more useful is at either end of the distribution curve, identifying those papers that are or are not likely to be most falsifiable.
Gardener Comments
Josh Randall:
This paper provides an intriguing proposal increasing the reproducibility and contentedness of what might otherwise be less penetrable domains of knowledge. The author provides two possible routes for falsifiability to be further included in the modern research program, a falsifiability statement and index. The statement relies on the author of a manuscript's knowledge of the theoretical and empirical basis of their claims and is only encouraged to maintain full honesty by the reviewers or some incongruence with a possible index score. The index score seems difficult to implement, liable to gaming if it were to be involved in scoring papers by search engines, and possibly serve as another form of discipline specific norms possibly limiting interdisciplinary engagement. The statement, while relying on the honesty or knowledge of unpaid reviewers, seems much more possible and an important practice in understanding how disciplines of knowledge are fundamentally limited. One point that I would like the author to specifically address is whether these statements are meant to explicitly name the disciplines for which results appear applicable and possibly serve as loci of falsifiability? For example, a claim about the development pattern of a leaf could be tested by checking against other species as a macroevolutionary trend, by testing the development over an individual's lifetime, by performing negative tests through genetic experiments, and by observing whether this trend is present in natural systems. Should authors set specific limits for their observations and what role would this play in either encouraging or discouraging interdisciplinary or subject focused research?
Mark (Senior scientist with a focus on meta science):
Overall, this is an interesting idea but one that could benefit a lot from more consideration. In particular while the proposed measurement mechanism seems well justified, it may not have done a great job at spotting some of the most critical examples of falsified science, e.g., situations where reasonably well designed experiments were used with slightly hand wavy statistics to argue points that stand up to scrutiny, but eventually are found to fail to replicate years later.
One thought on a way to extend the current work would be to discuss steps scientists could take to robustly their work to failure modes that are common in science that is eventually falsified. As a simple example, something like checking for pre-registration and checking that the pre-registered hypotheses are in fact those that are discussed in the work would be two reasonable and relatively objective rubric items. Many more in this style might make for an almost entirely objective measure for falsifiability while also helping scientists work out how to avoid pitfalls in their work.
Pierre Mercuriali:
This article presents a novel way of measuring the "quality", or how interesting a scientific publication may be, through its review. It is therefore very relevant to SoS' goals of proposing novel and interesting ways of doing scholarly work in particular. This measure is called the Falsifiability Statement (FS), and is to be written by the authors themselves.
One very interesting point is that the authors apply their FS proposal to their own paper. This is an excellent illustration of the process that has the advantage of both illustrating it and making the points clearer (and making the review easier).
I have two comments regarding the paper's own FS.
1. I see an additional limitation to the process: Feasibility: coming up with an FS is additional work on the researcher's part. Although the statements and concepts might be at the core of the scientific process in a paper, and therefore already into the mind of the writer, they still require additional work for which motivation is required. Though an interesting exercise, if the immediate reward is not clear, people might not be motivated enough to adopt a new practice.
I would suggest a tool to help researchers come up with these in a fast and harmonized manner, by providing them with, e.g., a taxonomy of assumptions, types of falsification, etc. and perhaps the most common pitfalls and falsifiable statements in research.
This would help create a "standard" for an FS, much like the "contributor's taxonomy"
https://credit.niso.org/
and answer limitation 4 (Adoption assumption).
2. Asking reviewers to come up with their own FS/limitations and aggregating the answers could provide a qualitative and machine-readable way of reviewing. Comparing the reviewers' and the authors' FS could lead to deeper insight into the quality of a paper.
Dr. Christian Thurn:
This is a very interesting and thought-provoking paper. I like the analogy to police investigations and that they need to state which evidence falsified their conclusions.
I miss a reference to the statement that "there are over 40 variants of the h-index". And maybe it is also worth mentioning that scite-ai is trying to implement a somewhat similar index in which it differentiates citations that support a statement from those that contradict a statement.
In the falsifiability statement I think it is not enough to say: "Falsified by: -qualitative attitude survey", or "falsified by: -empirical testing/research". Such falsifiability statements need to be done much more carefully: what would the attitude survey or the empirical research need to study with which sample and which effect would falsify the statement.
Now all the difficulties of the social sciences come in. Please set a good example and write more specifically how your statements can be falsified.
One meta-comment regarding replications: Is the original study or the replication falsifiable? Or both?
Dr. Payal B Joshi (PhD in chemical sciences):
The premise of the article is articulated in a manner to deviate from one index to another (F-index). Though the premise is not flawed, there are certain observations to the proposal that have been overlooked by the authors.
1. While designing f-index, we need to assess heterogeneity of authors and reviewers alike.
2. We have 2-dimensional indices such as i10 and citation counts besides h-index. Authors have only taken one metric for comparison which is skewed in my opinion. Authors can provide some insight for other research metrics too. In fact, i10 is rarely discussed and shall be great to provide critical information on the same.
3. Authorship order also influences the f-index, and for simplicity if only the first author is considered, it may not be of much utility.
Apart from the above limitations in the article, it also lacks mathematical equations to derive the index using an example. Though an honest attempt to discuss falsifiability seems good, it is not yet backed by sufficient clarity per se.
Authors have interestingly provided a FS statement for their own article. Thus, it serves as a primer. If authors can provide few examples of deriving them, shall make the scheme of their thoughts more in alignment to the objective.
Overall, the paper is of adequate length thus making for an engaging reading and must reach wider readership.
Mario Pasquato:
My main comment is that the falsifiability scores assigned by the author (self reported) and by the referee could be used together, represented e.g. as a point on a plane with one axis corresponding to each. I expect papers to cluster in this plane in a meaningful way. For instance, a high self-reported score with a high referee score would likely mean that the research is indeed falsifiable. High self reported and low referee report may mean that either the referee misunderstood the work or the author is overselling the falsifiability of his results, and so on. Whether these clusters form and how clear cut they are is an empirical question that depends on the scale used to measure falsifiability.
Anonymous1:
The current paper, on assessing falsifiability, was thought-provoking. Falsifiability is a core concern of empirical research methods, at least within many mainstream research traditions. However, while an important and complex issue, as extensively discussed already by Popper (see below), in principle every study published either does test a falsifiable (and therefore appropriately constrained) claim or is simply fatally flawed. It's therefore unclear what a falsifiability statement, in the form proposed in the current paper, would add to existing scientific norms.
As I read the paper, it seems to me to concern problems of limitations or generalization rather than falsification - e.g., whether a given paper addresses all relevant assumptions and, possibly, the extent of its implications. If the idea is that papers often overinterpret their results, or claim to falsify broad theoretical frameworks without sufficient justification, then I think this needs clarification.
I wavered between "yes" and "no" on publication given the above.
Yseult hb:
The paper is well written but I feel part of the argument is missing: why do we need a falsifiability index in the first place? There's no real development of that point which I would think is primordial. Why do we need another metric? Would that really help or serve its purpose? Given the many metrics we already have and their flaws and abuse, I remain highly skeptical about this new one. The paper would highly benefit from having an additional paragraph to answer the questions above. In its current shape, it doesn't bring much.
References
Basbøll, T. (2022) ‘Blog post: Inframethodology – The Automatic C’ https://blog.cbs.dk/inframethodology/
Brezis, E.S. and Birukou, A. (2020) "Arbitrariness in the peer review process". .Scientometrics.123 (1): doi:10.1007/s11192-
Brown, C. et al. (2008) ‘Automatic measurement of propositional idea density from part-of-speech tagging’. Behav Res Methods. doi.org/10.3758/brm.40.2.540
Derksen, M. (2019) ‘Putting Popper to work’. Theory & Psychology, doi.org/10.1177/0959354319838343
Hirsch, J.E. (2005) ‘An index to quantify an individual's scientific research output’. Proc Natl Acad Sci U S A. doi.org/10.1073/pnas.0507655102
Feyerabend, P. (1974). Against Method: Outline of an Anarchistic Theory of Knowledge. Humanities Press.
Lagakis, P. and Demetriadis, S. (2021) ‘Automated essay scoring: A review of the field,’ International Conference on Computer, Information and Telecommunication Systems (CITS), doi: 10.1109/CITS52676.2021.9618476.
Latour, B. and Woolgar, S. (1979) ‘Laboratory Life: The Construction of Scientific Facts (online preview), Princeton, New Jersey: Princeton University Press, 1986, ISBN 0-691-09418-7
Moed, H.F. (2014) ‘The Multidimensional Assessment of Scholarly Research Impact’, Informetric Research Group, Elsevier. https://arxiv.org/pdf/1406.5520.pdf
PLoS (Public Library of Science), (2022) research paper guidelines https://plos.org/publish/metrics
Popper, K. (1935) ‘The Logic of Scientific Discovery’ 2nd Edition published 2002 by Routledge ISBN 9780415278447
Rosy, J. (2020) ‘H-Index and Its Variants: Which Variant Fairly Assess Author’s Achievements’ Journal of Information Technology Research Volume 13 • Issue 1 • January-March 2020
Severin, A. et al. (2022) ‘Journal Impact Factor and Peer Review Thoroughness and Helpfulness: A Supervised Machine Learning Study’ preprint article doi.org/10.48550/arXiv.2207.09821
Thelwall, M. and Kousha, K. (2021) ‘Researchers’ attitudes towards the h-index on Twitter 2007–2020: criticism and acceptance’. Scientometrics 126, .doi.org/10.1007/s11192-021-03961-8
Zixuan, K. et al. (2018) ‘Learning to Give Feedback: Modelling Attributes Affecting Argument Persuasiveness in Student Essays’ Proceedings of the Twenty-Seventh International Joint Conference on Artificial Intelligence doi.org/10.24963/ijcai.2018/57
Zixuan, K. and Vincent, N. (2019) ‘Automated Essay Scoring: A Survey of the State of the Art’ Proceedings of the Twenty-Eighth International Joint Conference on Artificial Intelligence doi.org/10.24963/ijcai.2019/8