Attitudes Toward Artificial General Intelligence: Results from American Adults in 2021 and 2023
Authors: Jason Jeffrey Jones and Steven Skiena
Author: Jason Jeffrey Jones1 and Steven Skiena2
Date: February, 2024
Text: PDF (https://doi.org/10.53975/8b8e-9e08)
A compact, inexpensive repeated survey on American adults’ attitudes toward Artificial General Intelligence (AGI) revealed a stable ordering but changing magnitudes of agreement toward three statements. Contrasting 2021 to 2023, American adults increasingly agreed AGI was possible to build. Respondents agreed more weakly that AGI should be built. Finally, American adults mostly disagree that an AGI should have the same rights as a human being; disagreeing more strongly in 2023 than in 2021.
Introduction
Are we ready for in silico equals? Depending on who you choose to listen to, you will find arguments that this question is premature (Marcus, 2022) timely (Bubeck et al., 2023) or already too late (Yudkowsky, 2023). Here we present the results of a short, repeated survey on attitudes toward Artificial General Intelligence (AGI). We can say with confidence that (in our samples) American adults believe building an AGI is possible. Many (but not the majority) agree that AGI should be created. Most disagree with the idea that an AGI should have the same rights as a human being.
Prior Work
This study is not the only recent assessment of attitudes toward artificial intelligence. Sindermann et al. (2021) recently contributed the Attitude Towards Artificial Intelligence (ATAI) scale – a five-item scale measuring two factors: Acceptance and Fear. The ATAI comprised concise prompts such as “I trust artificial intelligence” and “Artificial intelligence will destroy mankind.” Across Chinese and German respondents, those who scored high in Acceptance expressed high willingness to use artificial intelligence systems (e.g. self-driving cars). Those who scored high on the Fear factor expressed lower willingness. Overall, on the 11-point Likert range, mean responses for both factors sat near the midpoint.
Similarly, Schepman and Rodway (2020) created a 32-item General Attitudes towards Artificial Intelligence Scale with two equally-sized subscales for positive and negative attitudes. In contrast to Sindermann et al., Schepman and Rodway emphasized the difference between attitudes and technological readiness (Lam et al., 2008). Technological readiness, they argued, predicts consumer decisions – e.g. will I buy and use a home smart speaker? However, artificial intelligence systems are more frequently deployed by organizations, not individuals, and the decision is made for the individual, not by the individual. Therefore, they argue it is important to measure general attitudes toward artificial intelligence and comfort with proposed applications (e.g. using facial recognition to automatically detect, identify and fine jaywalkers) to inform legislators and organizations. Overall, Schepman and Rodway found that positive attitudes were associated with respondents’ perceived utility of potential applications and negative attitudes with perceived malevolence (“I find Artificial Intelligence sinister”) or dystopian potential (“Artificial Intelligence is used to spy on people.”)
Neither of the above, and no other work we are aware of has specifically measured attitudes toward AGI specifically. Additionally, repeated surveys such as this one are rare. The current study contributes results on a novel facet of artificial intelligence attitudes and provides evidence toward temporal trends.
Methods
The data we collected and the analysis code we wrote to support this article are publicly available at https://osf.io/df2yx/. In March 2021 and again in April 2023, we gathered responses to three survey items regarding AGI. In the prompt, we defined AGI this way: “Artificial General Intelligence (AGI) refers to a computer system that could learn to complete any intellectual task that a human being could.” The survey items listed in Table 1 followed.
2021 Survey
We sought low-cost, high-volume respondent recruitment. After evaluating several alternatives, we selected Google Surveys (Sostek & Slatkin, 2017). At the time, Google Surveys provided a service to distribute one-item surveys to a representative sample of American adults (Keeter & Christian, 2012) for $0.10 per respondent. Self-report age and sex demographics were automatically appended to each response. The platform claimed to deliver samples representative across age, sex and geographic region, with the Census Bureau’s American Community Survey as the reference compared against.
In March 2021, we distributed three separate one-item surveys using the AGI definition from above and the items from Table 1. The platform selected respondents, sent a push notification inviting them to the survey and collected the response. We requested 300 respondents to each item and paid $90 total: $0.10 per item * 300 respondents * 3 items. In Table 2, one can see the Google Surveys platform over-delivered on quantity of respondents in each case; we believe this was due to the platform attempting to fulfill its promise to automatically adjust sampling to deliver a representative sample. Figure 1 depicts what a respondent would have seen.
2023 Survey
The 2023 survey was distributed on the Prolific Academic platform. We were unable to use Google Surveys, because the product was abandoned and shut down in November 2022. Prolific (2014) also provides distribution of online surveys to samples of American adults. (See Adams et al., 2020 for a recent evaluation of the platform.) It is important to note that on Prolific a premium is charged to target a representative sample, and we paid the premium to do so. The total cost for 459 completed responses was $988, or $2.15 each.
Unlike the 2021 survey, the 2023 participants responded to all three items. The three items consisted of the same statements from Table 1. A seven-point Likert scale was again the set of possible responses, however, each point received its own verbal label. Figure 2 depicts what a respondent would have seen. The survey was created as a Qualtrics XM “Matrix table.” (In 2021, we had separated each item into its own administration only because that delivered the best cost-to-response ratio due to the affordances of Google Surveys). In 2023, because the entire sample responded to all three items, we were able to perform a correlational analysis (see Discussion) that was not possible for the 2021 survey.
Sample Demographics
In Table 2, we present the total count of respondents per sample, and percentages per Sex and Age category (On both platforms, only values of Female and Male were provided for Sex). Recall that each item was fielded to a separate sample in 2021, while in 2023 all participants responded to all three items simultaneously.Table 2. Demographics of each sample.
Results
We began with the simplest analysis. Using only the most recent data (viz. the 2023 Prolific sample), we contrasted the average response for all three items. We make the typical assumption of a Likert scale, and assign each of the seven responses a number from -3 to +3. Mean responses for each item are plotted in Figure 3.
Averages can sometimes distort the truth, and some scholars would object to mapping categorical responses to numerical values. For those reasons, we also present histograms containing the raw frequency of each categorical response here as Figure 4.
Next, we turn to a temporal analysis; we will contrast the magnitude of agreement for each item between the 2021 and 2023 samples. We make some assumptions to do so. First, we assume the samples are comparable. Second, we assume the Likert-scale responses can be mapped to equally-spaced numeric intervals of -3 for Strongly disagree through +3 for Strongly agree. Finally, we assume the platform effects (i.e. Google Surveys versus Prolific) are negligible. With these assumptions, we plot the change over years in Figure 5.
Welch two sample t-tests (which adjust degrees of freedom to account for unequal variance) indicated that the 2021 to 2023 differences for Possible to build and Same rights as a human were statistically significant with p < 0.001. The difference for Should be built (p = 0.10) did not reach the conventional criterion for statistical significance.
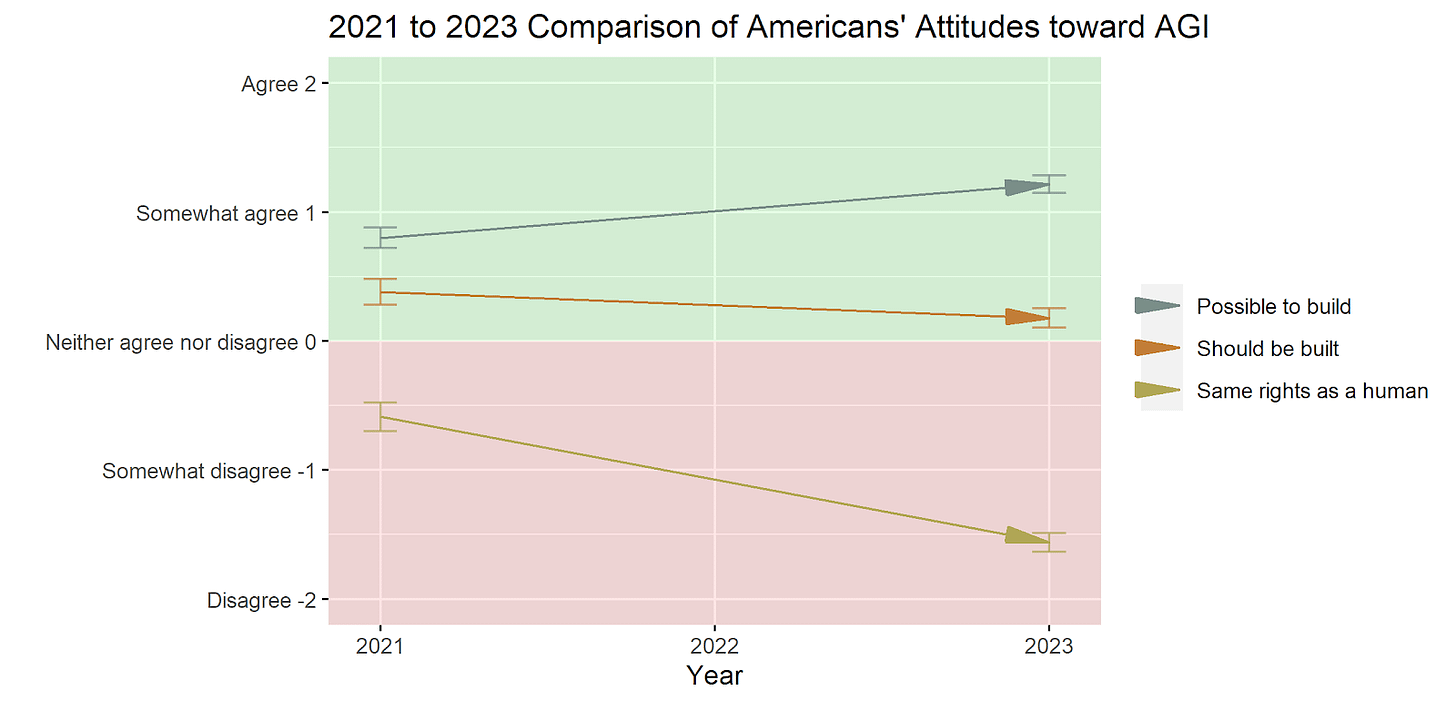
Finally, we explore potential differences across age and sex demographics. Figure 6 plots mean responses per age category. (In the Google Surveys data, only a bin is reported for each respondent. We mapped the age in years variable available in the Prolific sample to the same bins.) Statistical analysis (generalized linear models estimating Response from Age * Year) confirms visual inspection of the plots: agreement differs by year of sample for Possible to build and Same rights as a human as discussed above, and the strongest association with age is more disagreement from older adults for Same rights as a human in 2023.
Figure 7 plots 2021 and 2023 responses by sex. Both groups’ results conform to the patterns in Figure 5: increasing agreement for Possible to build; little change for Should be built and more severe disagreement for Same rights as a human. In a linear regression, the interaction between Sex and Year was statistically significant for Same rights as a human.
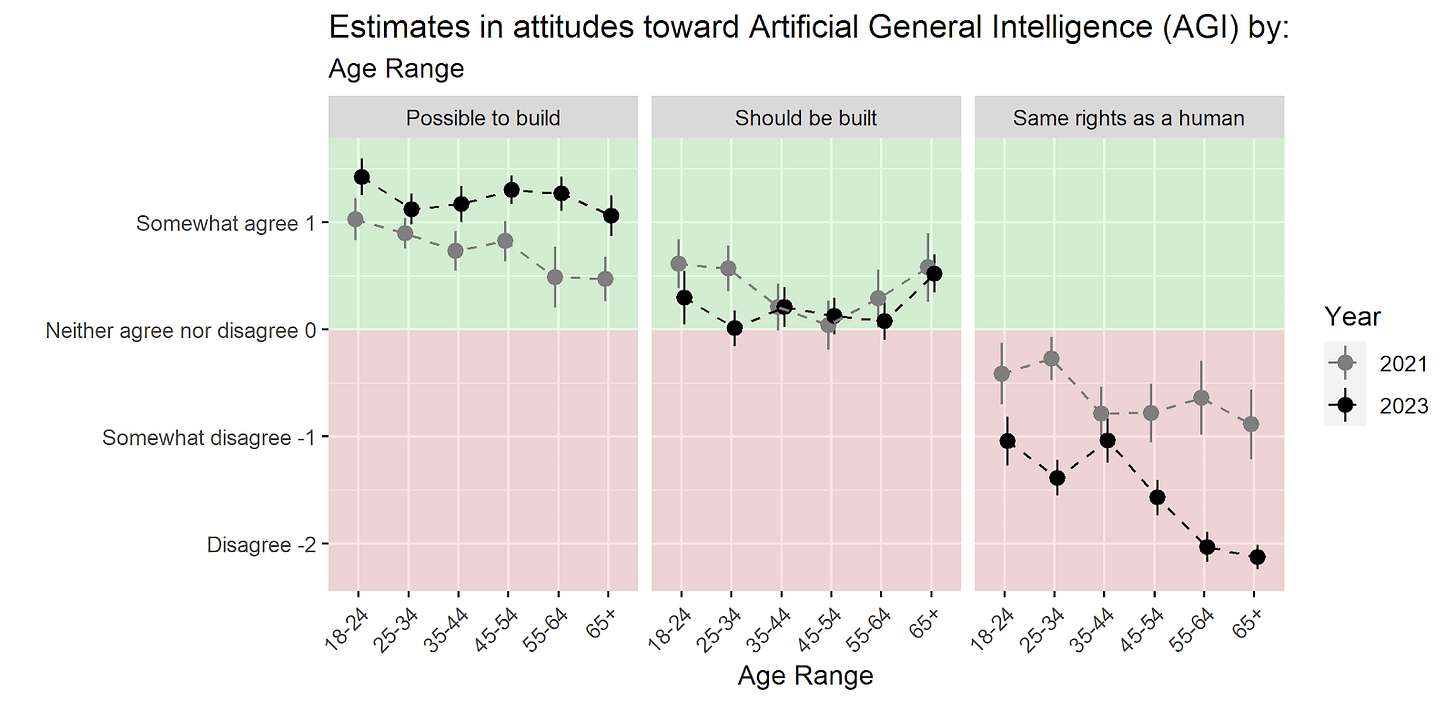
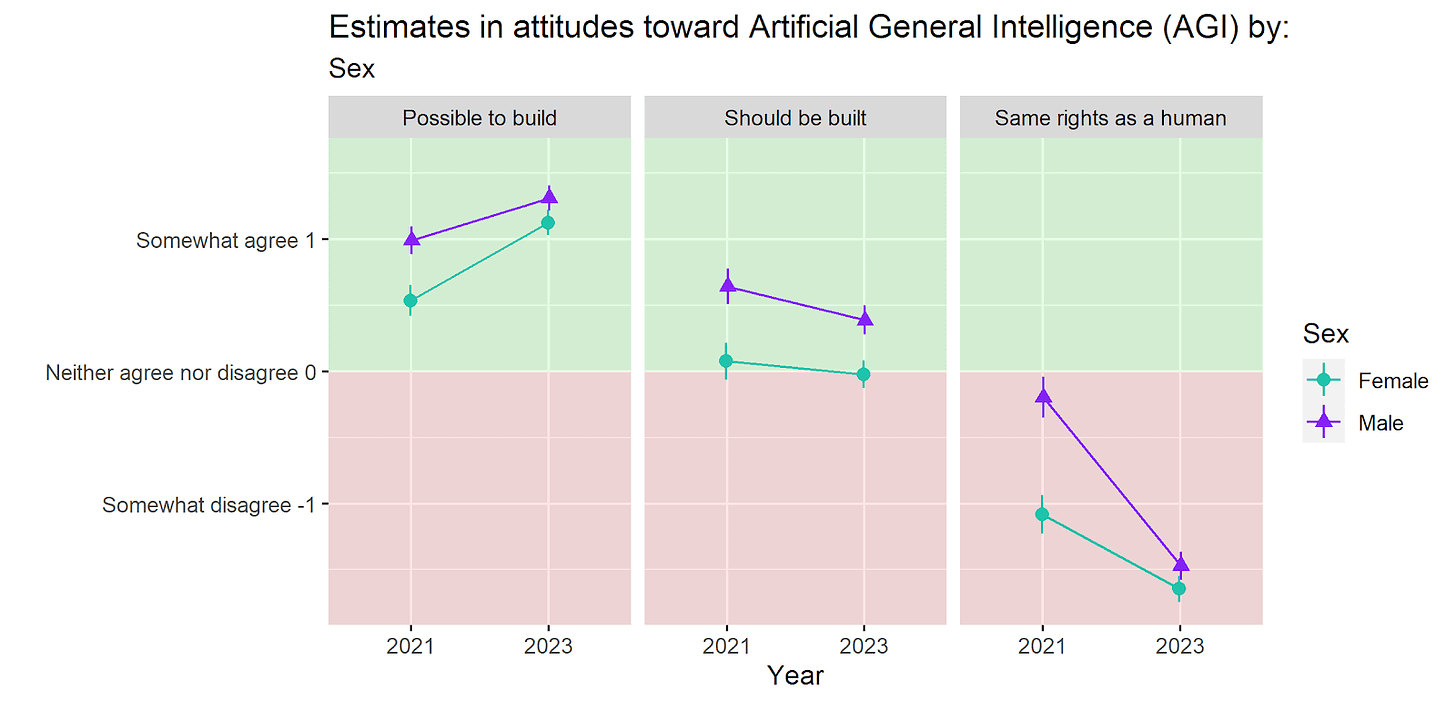
Discussion
Artificial General Intelligence – by our chosen definition – is not here yet. But American adults increasingly believe it is possible to build. Their agreement that AGI should be built is not increasing at the same rate. Their willingness to endorse equal rights for an AGI is trending in the opposite direction. As artificial intelligence systems become ubiquitous (as they are currently poised to) persistent, consistent measurement of these attitudes bears consideration.
We examined the relationship between attitudes and age (and attitudes and sex) not because we had theory or even a hypothesis to test. Instead, we explored the data for the same reason George Mallory gave for climbing Mt. Everest: “Because it’s there.” We urge readers to not overinterpret the results. Instead, note that across every demographic category, the trajectory of attitudes over time is consistent in direction. This is not a landscape of crossover interactions. There is a hint that males and older adults are souring to the idea of AGI peers faster than their (human) counterparts. Future scholars could follow up on that idea. However, we urge future surveys to attempt to discover correlates more predictive of AGI attitudes than mere demographics.
More generally, we argue that in the current moment, temporal trends at the aggregate level are more likely to be interesting than static subsample analyses. The capabilities of AI are developing rapidly, and public opinion toward its applications is likely also in flux. We understand the convenience of demographic subsample analyses – the marginal cost is only one more line of R code. Repeating a survey, on the other hand, roughly doubles the amount of time and effort of the research project. It is our hope that more researchers accept that burden, however. Compact, inexpensive, repeated surveys such as this one are an example we hope others follow. We will repeat this survey again in the spring of 2024 using the same methods as the 2023 administration.
For the 2023 survey only, every respondent encountered all three items. Thus, we can explore the associations between attitudes. Figure 8 contains boxplots for each pair of items. The thick black horizontal line in each marks the median response; blue diamonds mark the mean response. Responses for all items were positively correlated. Respondents who were more skeptical about the possibility of AGI disagreed that it should be built (r = 0.37, CI = [0.29, 0.45], p < 0.001). This makes intuitive sense. If you believe AGI is not possible, presumably you also believe it would be a waste of time to try to build one. Those who were more skeptical about the possibility of AGI also more strongly disagreed that it should have the same rights as a human (r = 0.13, CI = [0.03, 0.21], p = 0.007). Note, however, that human rights for AGI is unpopular across the spectrum of belief in its possibility.
Agreement that AGI should be built is associated with less disagreement that AGI should have the same rights as a human (r = 0.30, CI = [0.21, 0.38], p < 0.001). On average, those who support building AGI are against granting them rights, but less so than those against building AGI. Indeed, among those disagreeing that AGI should be built, lack of support for rights was nearly unanimous.
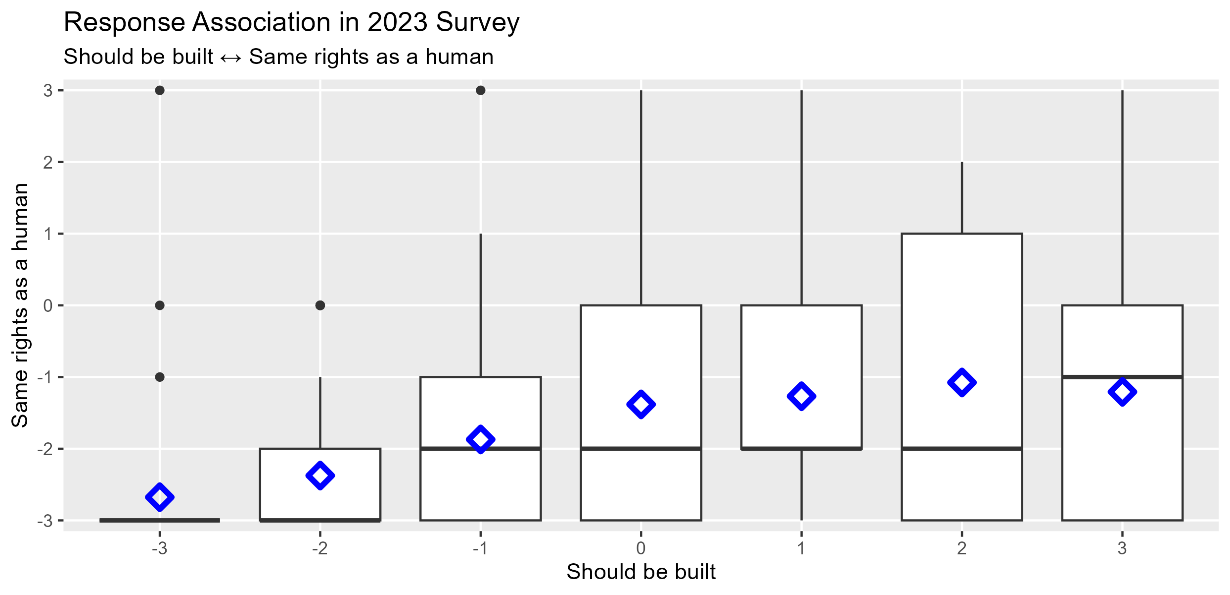
Agreement that AGI should be built is associated with less disagreement that AGI should have the same rights as a human (r = 0.30, CI = [0.21, 0.38], p < 0.001). On average, those who support building AGI are against granting them rights, but less so than those against building AGI. Indeed, among those disagreeing that AGI should be built, lack of support for rights was nearly unanimous.
Limitations
We present these results based on data we have instead of the ideal data we might wish we had. Ideal data collection would certainly have used the same platform. It was not our choice for Google to abandon their survey product, but perhaps we should have foreseen such. In any case, respondent recruitment method and survey platform are unavoidably confounded with time in our data.
It might have been useful to collect data at finer temporal resolution. One strength of the current interval is that it straddles an extremely salient news event in artificial intelligence – namely, OpenAI’s public release of ChatGPT. Given the investment and interest sparked by the recent generative AI wave, we expect AGI speculation and discussion will be increasingly salient to the general public. Future data will provide more clarity on the possible trends and associations explored here.
Conclusions
Like all responsible scholars, we disclaim the idea that these results are definitive or comprehensive. Americans’ attitudes toward AGI and artificial intelligence more generally deserve and are gaining increasing attention (O’Shaughnessy et al., 2023; Zhang & Dafoe, 2019). However, to our knowledge, these are the first results from a repeated survey regarding AGI fielded to national, representative samples. Thus, they can provide a reference point for further investigation. We take the liberty here to make the following recommendations: Large, carefully sampled, well-resourced surveys such as the General Social Survey, American National Election Studies and the World Values Survey should add and continue to field an item or scale specifically regarding AGI. Independent of these projects, scholars can and should make use of inexpensive, representative online survey platforms to consistently and persistently measure attitudes toward technology.
Gardener Comments
Andrew Neff (PhD in neuroscience):
Really interesting, I recommend publication, I just came here to leave a small recommendation about Figure 5-7 related to presentation. As it is, the Y-axis range does not capture the entire range, which could lead readers to overestimate the differences that were observed.
Joe R:
Overall this struck me as an excellent paper. The (mundane, not statistical) significance of the results seems dubious, but I'm tempted to recommend this paper for its impressive dedication to accuracy, brevity, and clarity.
Pros:
A good introduction succinctly summarizing results.
Publicly accessible data.
A clear and legible explanation of methods.
Simple, legible, appropriate graphics.
A commitment to repeat the survey in 2024.
Open acknowledgement of flaws.
Cons:
Simple, general questions that could be measuring lots of different things (like a general halo or horns effect of AI news).
A relatively small sample for a survey of national relevance (~300). But still respectable.
The inherent unreliability of survey data. (Acknowledged by the authors).
I'm honestly not quite clear on how useful these data will be to researchers. I agree with the authors that we shouldn't read too much into the results. But for ~$1k/year it is probably a cheap test worth doing. And having been done, it should probably be shared. Kudos to the authors for one of the best write-ups I've seen so far.
C:
I like the blunt honesty that the authors use with regard to their non-existing hypotheses. I also greatly value that they made their assumptions clear. However, I am hesitant for the following reasons:
Unclear how large the 2021 sample was. Unclear how sample size was determined
2021 was on a star-rating, in 2023 all items had labels. Why did you choose this different scale structure when implementing it in Qualtrics? And how did this potentially affect the results?
I agree that longitudinal studies are necessary, but I hope that more researchers follow panel-designs, in which they survey the same people repeatedly. This controls for a lot of unobserved variables and maybe you could consider a panel design for your study as well
Dr. Payal B. Joshi:
The article is relevant to the present trends on artificial intelligence. While there is a plethora of information pertaining to the topic that ranges from technological applications to mystical uses, the present study attempts to explore a lesser-known terrain on attitudes towards AI. The survey results are critically analyzed and limitations of the study are presented. Maybe one aspect that seems skipped by the author is - the study is based on American people's attitudes only: Can the results be entirely extrapolated to other parts of the world? Not quite. Also, I found a few polarized responses a little obvious that respondents provided therein - hence a detailed survey (as already highlighted to be conducted by author is mentioned) shall serve the purpose.
Overall, the paper is well-presented and thus I highly recommend publishing the paper as it is.
Thomas Gladwin (PhD):
I enjoyed reading this paper, on a clearly topical issue. It should help inspire future research - for instance, would opinions change from before to after watching the Star Trek trial of Commander Data? I appreciated the transparency on methods and the research process, as well as the creative and clear visualizations.
A minor comment: "We make the typical assumption of a Likert scale, and assign each of the seven responses a number from -3 to +3." and "some scholars would object to mapping categorical responses to numerical values." It's great that this is touched on, but maybe it would be helpful to make explicit what the "typical assumption" is and provide a reference to a paper on the issue? (E.g., for a counterargument to the "against" position", https://link.springer.com/article/10.1007/s10459-010-9222-y.)
References
Adams, T., Li, Y., & Liu, H. (2020). A Replication of Beyond the Turk: Alternative Platforms for Crowdsourcing Behavioral Research – Sometimes Preferable to Student Groups. AIS Transactions on Replication Research, 6(1). https://doi.org/10.17705/1atrr.00058
Bubeck, S., Chandrasekaran, V., Eldan, R., Gehrke, J., Horvitz, E., Kamar, E., Lee, P., Lee, Y. T., Li, Y., Lundberg, S., Nori, H., Palangi, H., Ribeiro, M. T., & Zhang, Y. (2023). Sparks of Artificial General Intelligence: Early experiments with GPT-4 (arXiv:2303.12712). arXiv. https://doi.org/10.48550/arXiv.2303.12712
Keeter, S., & Christian, L. (2012). A Comparison of Results from Surveys by the Pew Research Center and Google Consumer Surveys. https://www.pewresearch.org/politics/2012/11/07/a-comparison-of-results-from-surveys-by-the-pew-research-center-and-google-consumer-surveys/
Lam, S. Y., Chiang, J., & Parasuraman, A. (2008). The effects of the dimensions of technology readiness on technology acceptance: An empirical analysis. Journal of Interactive Marketing, 22(4), 19–39.
Marcus, G. (2022, July 1). Artificial General Intelligence Is Not as Imminent as You Might Think. Scientific American. https://www.scientificamerican.com/article/artificial-general-intelligence-is-not-as-imminent-as-you-might-think1/
O’Shaughnessy, M. R., Schiff, D. S., Varshney, L. R., Rozell, C. J., & Davenport, M. A. (2023). What governs attitudes toward artificial intelligence adoption and governance? Science and Public Policy, 50(2), 161–176.
Prolific. (2014). https://www.prolific.co/academic-researchers
Schepman, A., & Rodway, P. (2020). Initial validation of the general attitudes towards Artificial Intelligence Scale. Computers in Human Behavior Reports, 1, 100014.
Sindermann, C., Sha, P., Zhou, M., Wernicke, J., Schmitt, H. S., Li, M., Sariyska, R., Stavrou, M., Becker, B., & Montag, C. (2021). Assessing the attitude towards artificial intelligence: Introduction of a short measure in German, Chinese, and English language. KI-Künstliche Intelligenz, 35, 109–118.
Sostek, K., & Slatkin, B. (2017). How Google Surveys Works. http://g.co/surveyswhitepaper
Yudkowsky, E. (2023, March 29). Pausing AI Developments Isn’t Enough. We Need to Shut it All Down. Time. https://time.com/6266923/ai-eliezer-yudkowsky-open-letter-not-enough/
Zhang, B., & Dafoe, A. (2019). Artificial intelligence: American attitudes and trends. Available at SSRN 3312874.
Jason Jeffrey Jones is a computational social scientist whose expertise includes online experiments, social networks, high-throughput text analysis and machine learning. He is interested in humans’ perceptions of themselves and the developing role of artificial intelligence in society. He is an Associate Professor in the Department of Sociology and the Institute for Advanced Computational Science at Stony Brook University (corresponding author: jason.j.jones@stonybrook.edu).
You can support continuing surveys by donating to Jason Jeffrey Jones at https://www.buymeacoffee.com/jasonjeffrc. Dr. Jones will personally match each donation dollar-for-dollar up to $1500 per year. All funds will be spent deploying nationally-representative-sample surveys and paying the respondents.
Steven Skiena's research interests include the design of graph, string, and geometric algorithms, and their applications (particularly to biology). He is the author of five books, including "The Algorithm Design Manual" and "Calculated Bets: Computers, Gambling, and Mathematical Modeling to Win". He is Distinguished Teaching Professor of Computer Science, Director of the Data Science Laboratory and Director of the Institute for AI-Driven Discovery and Innovation at Stony Brook University.
I doubt the majority of respondents are familiar with what they “think “ is AI versus the current batch of generative platforms plagued with the same biases of their creators. I am conflicted mainly by the lack of intellectual honesty and the lack of understanding, especially by regulators. The world will get there. It’s more a question of will it be used to conquer others, or will we be watering crops with Kool-aid and wondering why nothing grows.
I am not at all worried about AGI doing our work for us. I am extremely worried about the accelerating trend to allow AGI to do our thinking for us.